Aaron Zweig
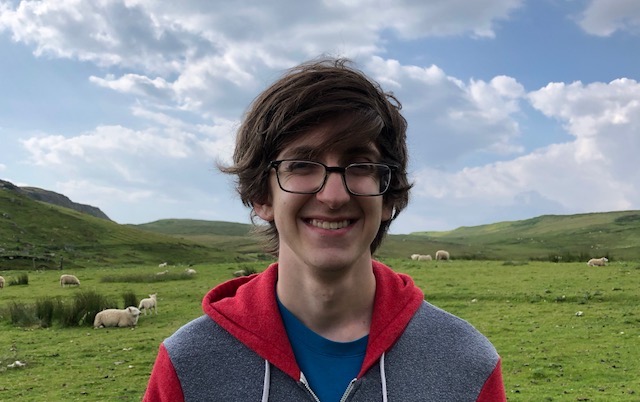
I am a Postdoctoral Research Associate, jointly appointed in the Knowles lab at the New York Genome Center and the Azizi lab. I’m currently working on applying methods from geometric deep learning to the analysis of spatial transcriptomic data and inference of gene regulatory networks.
Recently, I completed my PhD in the CILVR group at NYU, advised by Joan Bruna.
My thesis primarily concerned the theoretical properties of symmetric and anti-symmetric neural networks. During my PhD I studied quantitative approximation bounds based on pairwise attention in symmetric architectures, characterized symmetric functions that are provably learnable with gradient methods, and analyzed the capacity to approximate symmetric functions defined over infinitely large sets.
Previously, I’ve worked on graph neural network autoencoders, reinforcement learning theory, and differentiable approximations to discrete operators. My full CV is available here, feel free to reach me at az831@nyu.edu